Inferential Data Analysis Techniques
are pivotal in comprehending data patterns and making future predictions. It is an advanced methodology that helps us make assumptions about a population from a sample, test hypotheses and make informed decisions in an uncertain environment. Its importance is recognized in various fields like science, business, and technology.
The Fundamentals of Inferential Statistics
consist of two major elements: estimation and hypothesis testing. Estimation empowers us to infer the population parameters from the sample data while hypothesis testing guides us in making decisions and conclusions about the population.
The Role of Sampling and Sample Distributions
is significant in inferential analysis. Understanding how varying samples can lead to different estimations and how sampling distributions function is crucial for accurate inferential statistics.
Significance Testing and P-Values:
Tests such as Chi-square, t-tests, and ANOVA are used to ascertain whether a result is likely due to chance or there is a statistically significant effect or difference. The p-value plays a critical role here, providing a measure of probability that assists in decision making.
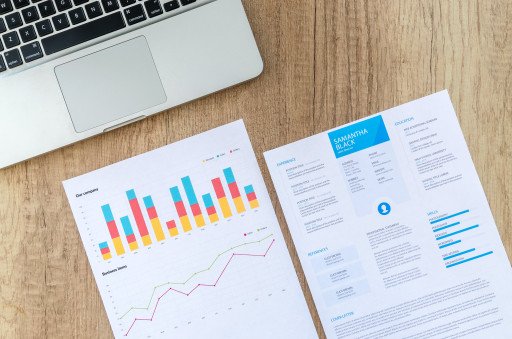
Confidence Intervals and Precision Estimation:
Confidence intervals present a range of values for our population parameter, rather than point estimates. They offer a range within which the true value of the parameter is likely to lie, providing us with a more refined understanding of the population.
Predicting Outcomes with Regression Analysis:
Regression models are potent tools for predicting and explaining outcomes. From simple linear regression to multiple regression, these models aid in deciphering complex relationships between variables and making accurate predictions about future events or trends.
Master Excel Cohort Analysis Detailed Guide can further enhance your understanding in this field.
Analysis of Variance (ANOVA):
ANOVA is a statistical method employed to compare means and comprehend differences between groups. This technique provides deep insights into the data, whether it’s analyzing a single factor with a one-way ANOVA or complex designs with a multi-way ANOVA.
Non-parametric Tests for Unmet Assumptions:
Not all data meets the assumptions required for traditional parametric tests. Here, non-parametric tests like the Mann-Whitney U test or the Kruskal-Wallis H test offer an alternative path for analysis without those strict assumptions.
The Chi-Square Test for Independence is a non-parametric test used to determine if there’s a significant association between categorical variables. Its results help understand whether variables are related or independent of each other.
In conclusion, mastering Inferential Data Analysis Techniques is indispensable for any data-driven professional. With these techniques, one is better equipped to uncover truths hidden within the numbers and make sense of the world through data.
Related Posts
- 5 Essential Data Analytics Tools Guide for Enhanced Business Insights in 2023
- Real-Time Data Analysis: 5 Benefits of Ad Hoc Reporting for Businesses
- 10 Crucial Steps to Master Excel Cohort Analysis: A Detailed Guide
- Mastering Time Series Analysis in Excel: A 10-Step Guide
- 7 Key Techniques to Master Primary Data Analysis